AI Governance: It’s More Than Following the Rules, It’s About Taking Responsibility
By Marvin Hanisch, Associate Professor, Faculty of Economics and Business – University of Groningen
Artificial Intelligence (AI) is changing how tasks are performed across many fields, but this technological revolution demands careful governance. As organizations assess and integrate the diverging regulatory paths of the EU, the US, and China, one message is clear: robust AI governance is a strategic imperative, irrespective of legal mandates. This article highlights the difference between AI governance and compliance and offers practical insights from our research to help organizations build governance frameworks that align with their strategic objectives and organizational values.
Governance vs. Compliance: Understanding the Difference
With the EU stepping up its AI regulation through the AI Act, the US and China are scaling back on regulation or adopting a more lenient approach to stimulate AI industry growth. The related public discourse frequently emphasizes the associated tradeoff between speed and safety. On the one hand, rapid innovation cycles drive competitive advantages and technological breakthroughs. On the other hand, careful testing and reflecting on unintended consequences provide the basis for long-term viability and public trust.
AI governance is more than regulatory compliance—it is a strategic choice that needs to align with organizational goals and values. Companies with strong governance frameworks can innovate responsibly, earn stakeholder trust, mitigate long-term risks, and thereby create a competitive advantage.
In light of these regulatory differences, it is critical for organizations to recognize that regulatory compliance is only a starting point and that they must establish their own policies for developing and using AI. Regulations can provide guidance, but they are not, and should not be, a substitute for AI governance. In fact, AI governance not only interprets compliance rules within the organization’s specific context but also creates internal mechanisms to ensure that AI development and usage align with the organization’s goals and values. This extends far beyond mere regulatory compliance. Specifically, effective AI governance focuses on three layers of control:
- Input control: Checks the composition, integrity, representativeness, and license of the data used in AI models.
- Throughput control: Validates the documentation, understandability, openness, and accessibility of the algorithms.
- Output control: Assesses systematic bias, accuracy, efficacy, and user response in relation to predictions.
Importantly, organizations must proactively create governance structures that integrate AI into strategic objectives and define the normative bedrock on which it operates. For example, a financial institution using AI to assess credit risk might prioritize accurate and unbiased outputs to minimize loan defaults. In contrast, a health insurer might emphasize input and throughput controls to give patients the confidence to share sensitive data. Once the goals and values are defined, organizations can formulate policies on how AI systems are designed, deployed, monitored, and adjusted over time.
Residual Task Responsibility: The Core of AI Governance
What our research and interviews with executives reveal is that one of the most difficult challenges in AI governance is the assignment of “residual task responsibility.” AI itself cannot assume responsibility because it does not possess legal personhood, which raises a latent concern about accountability when AI is involved in tasks. For example, when an autonomous vehicle crashes, who is responsible—the software engineers, the manufacturer, or the user? The same dilemma applies to AI-driven hiring tools that produce biased results or financial models that make flawed investment decisions.
Defining accountability is especially complex as AI systems become more autonomous. As long as humans are “in the loop,” the residual task responsibility typically rests with the human who either defines the tasks or executes tasks assigned by the AI. However, the situation gets critical once AI becomes more agentic and human involvement is reduced to a minimum. In this scenario, defining the task scope becomes essential to ensure the AI operates within clearly defined bounds that are transparent to those affected, allowing humans to retain control over whether and how they wish to engage in AI-driven tasks.
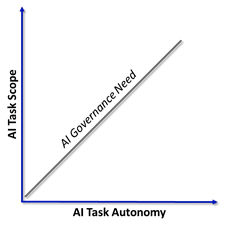
Implementing AI Governance in Practice
In practice, AI governance involves difficult tradeoffs, and our research identified several key considerations, such as the following:
- Centralization vs. Decentralization: This considers whether AI governance should be managed by a central authority within the organization or distributed across various teams or business units. Centralization ensures consistency and standardization, while decentralization allows for flexibility and tailored approaches to specific AI use cases.
- Internalization vs. Externalization: This centers on whether an organization develops and owns AI models internally or relies on external providers. Internalization offers greater control and transparency, whereas externalization can reduce costs and development burdens. However, externalization may come with higher governance demands and potential risks to privacy and security.
- Promotion vs. Prevention: This involves balancing the encouragement of AI experimentation with the regulation of its use to prevent unintended consequences. A promotion focus fosters fast adoption and learning, while a prevention focus emphasizes constraining guidelines and policies.
There is no definite right or wrong answer to these tradeoffs, and organizations must define their priorities. Those operating in highly regulated sectors, such as finance and healthcare, may need stricter oversight, while organizations in fast-moving technology sectors might prioritize flexibility. Understanding industry norms and the specific AI use case can help organizations develop a suitable governance framework tailored to their needs.
Defining Governance Structures: Key Questions for AI Leaders
To build an effective AI governance framework, there are a few fundamental questions to consider:
- Why is governance necessary—compliance, risk mitigation, reputation management?
- How should governance be structured—policy-based, risk-driven, or values-led?
- Who makes governance decisions—executives, compliance teams, external advisors?
- Where does governance apply—specific use cases, business units, entire organizations?
- When should governance be enforced—throughout the AI lifecycle with regular audits or only at key checkpoints?
- What aspects require governance—data use, model risks, output bias?
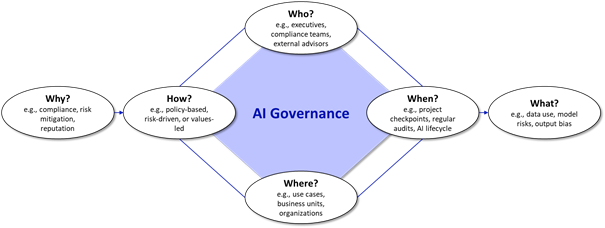
Conclusion: Governance as a Competitive Advantage
AI governance is more than regulatory compliance—it is a strategic choice that needs to align with organizational goals and values. Companies with strong governance frameworks can innovate responsibly, earn stakeholder trust, mitigate long-term risks, and thereby create a competitive advantage. The first step is recognizing AI governance as a cross-functional responsibility that centers on strategy and spans IT, legal, and operations. Organizations that treat AI governance as a strategic function rather than a compliance burden will be better positioned to prioritize their AI activities and deploy resources effectively.
Reference:
Hanisch, M., Goldsby, C. M., Fabian, N. E., & Oehmichen, J. (2023). Digital Governance: A Conceptual Framework and Research Agenda. Journal of Business Research, 162, Article 113777, 1–13. https://doi.org/10.1016/j.jbusres.2023.113777