Generative AI: Revolutionizing Supply Chains in the Digital Age
By Phani Kambhampati, Senior Director – Enterprise Data, AI, and Digital Strategy, UPS
In today’s dynamic business landscape, characterized by global competition, fluctuating consumer demands, and potential disruptions, optimizing supply chains, enhancing efficiency, and ensuring resilience is no longer a choice but a strategic imperative for ensuring business continuity and growth. Generative AI (GenAI) is transforming intelligent automation and data-driven decision-making. This technology surpasses traditional AI by its ability to analyze data and generate new information, presenting a strategic advantage for supply chain leaders. However, unlocking the true potential of GenAI hinges on one crucial element: high-quality, well-managed, and trusted data.
Unlike conventional AI, which focuses on classification and prediction, GenAI delves deeper. It learns the intricate relationships within data, enabling it to:
- Generate highly granular demand forecasts: Moving beyond historical trends, GenAI can incorporate real-time data, including weather patterns, social media sentiments, and external market shifts, resulting in dynamic and adaptable forecasts crucial for optimizing inventory levels and production schedules. Accurate forecasts heavily rely on the quality and completeness of data.
- Revolutionize inventory management: GenAI surpasses static safety stock calculations. It recommends optimal inventory levels, minimizing stockouts, and reducing holding costs by analyzing vast datasets encompassing historical sales, consumer behavior, and supplier lead times. UPS, a leader in supply chain innovation, actively explores AI-powered solutions to optimize various aspects of its operations, including inventory management. For instance, leveraging historical data, AI can help predict future product demand better, enabling optimized warehouse stocking levels based on predicted demand patterns.
- Orchestrate production with laser focus: Production planning has always been an intricate dance. GenAI analyzes production capacity, resource constraints, and order priorities to generate dynamic production plans and schedules. This ensures efficient resource allocation, eliminates bottlenecks, and expedites product delivery. Companies like UPS are actively exploring how GenAI can optimize routing and scheduling for their vast delivery network.
- Proactive maintenance for a resilient supply chain: Companies cannot wait for equipment failures before fixing them, nor can they spend their revenues on maintaining a schedule. GenAI solves this challenge by analyzing sensor data and historical equipment performance and predicting potential breakdowns, enabling proactive maintenance interventions. This approach minimizes costly downtime, safeguards uninterrupted production processes, and helps save money by avoiding expensive repairs or replacements.
- Weave sustainability into the supply chain DNA: Not only does GenAI help optimize inventory management and enable just-in-time equipment maintenance, but GenAI can also foster eco-conscious practices. GenAI helps analyze factors like material usage, transportation routes, and packaging solutions to identify areas for environmental improvement. This empowers businesses to embed sustainability throughout their supply chains.
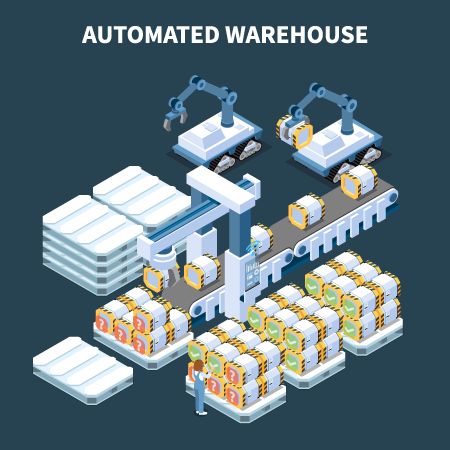
In conclusion, GenAI is a game-changer poised to revolutionize the very fabric of supply chain management. By harnessing the combined strengths of human expertise and GenAI, organizations can navigate the complexities of the modern supply chain landscape.
Challenges and Concerns: Embracing AI Responsibly
While GenAI offers a promising future for supply chain optimization, it’s crucial to acknowledge the potential challenges and ethical considerations accompanying this powerful technology.
- The Black Box Conundrum: Unlike traditional rule-based AI, GenAI’s decision-making process can be opaque, making understanding the rationale behind its outputs challenging. This lack of transparency can lead to reduced trust and limited ability to debug.
Explainable AI (XAI) techniques are actively being developed to address this issue. XAI aims to shed light on the internal workings of AI models, offering deeper insights into the decision-making processes of GenAI models and fostering greater trust and transparency.
- Ethical Considerations: Although many ethical aspects need to be considered, bias in AI models is one of the foremost. Typically, this bias is caused by bias in the underlying data used to train GenAI models. The model can inadvertently perpetuate biases present in the training data, leading to discriminatory or unfair outcomes.
Mitigating bias requires implementing robust data governance practices and employing diverse datasets during model development, which are crucial. Additionally, ongoing monitoring and evaluation of AI models are essential to detect and address potential biases.
- Integration with Other Technologies: The true benefit of GenAI will require integration with other cutting-edge technologies like blockchain and the Internet of Things (IoT) to create a more intelligent and interconnected supply chain ecosystem. Imagine a scenario where:
- Blockchain ensures secure and transparent data exchange throughout the supply chain.
- IoT sensors offer real-time information on inventory levels, equipment status, and environmental conditions.
- GenAI leverages this combined data to optimize processes, predict disruptions, and make real-time adjustments for enhanced efficiency and resilience.
- The level and depth of integration needed to make this possible, enabling the next generation of GenAI models, is complex and in its infancy.
Data: The Cornerstone of Success
While GenAI offers a powerful toolkit, like conventional AI, it is only as effective as the data on which it is trained. High-quality, well-managed, and trusted data is the cornerstone of successful GenAI implementation. To ensure fit-for-use data is available to train GenAI models, organizations must prioritize:
- Data Quality: High-quality data is paramount. Inaccurate, incomplete, or inconsistent data can lead to skewed results and unreliable model outputs. Implementing robust data governance practices ensures data accuracy throughout the supply chain ecosystem. This includes establishing clear data ownership, standardization of data formats, and regular data cleansing procedures.
- Data Granularity: The more granular the data, the richer the insights GenAI can generate. Going beyond basic sales figures, incorporating data points like customer demographics, product-specific details, and real-time inventory levels empowers GenAI to provide highly accurate forecasts and optimize processes with greater precision.
- Real-time Integration: Seamless integration between GenAI and various data sources across the supply chain is essential for genuinely dynamic decision-making. This allows GenAI to factor in real-time data like weather patterns, traffic conditions, and social media trends, enabling proactive adjustments and fostering greater supply chain agility.
- Data Security: Protecting sensitive data from unauthorized access or manipulation is crucial. Implementing robust cybersecurity measures safeguards data integrity and fosters trust in the information used by GenAI models. This includes employing encryption techniques, access controls, and regular vulnerability assessments.
- Data Governance Framework: Having a comprehensive data governance framework in place is essential for managing data organizationally and effectively. This framework should encompass policies and procedures for data collection, storage, access, and usage. Additionally, it should address data ownership, accountability, and compliance with relevant regulations.
- Data Integration: Seamless integration between GenAI and existing enterprise systems ensures data flows smoothly, enabling real-time decision-making.
- Data Lakes: GenAI thrives on vast amounts of data. Data lakes serve as centralized repositories for storing this data, encompassing structured, semi-structured, and unstructured information from diverse sources across the supply chain ecosystem. This readily available data pool empowers GenAI models to learn from a broader range of information and generate more comprehensive insights.
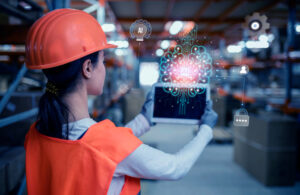
Beyond these core aspects, fostering a data-driven culture within the organization is vital. This involves:
- Encouraging data literacy: Equipping employees with the skills to understand, interpret, and utilize data effectively is crucial for maximizing the value derived from GenAI.
- Promoting data sharing: Breaking down data silos and fostering a collaborative environment where data is readily shared across departments allows for a more holistic view of the supply chain and empowers GenAI with a richer data pool for analysis.
- Continuous monitoring and improvement: Data quality and model performance require ongoing monitoring and evaluation. Regularly assessing data accuracy, identifying potential biases, and refining GenAI models based on real-world results are essential for ensuring the system continues to deliver optimal outcomes.
In conclusion, GenAI is a game-changer poised to revolutionize the very fabric of supply chain management. By harnessing the combined strengths of human expertise and GenAI, organizations can navigate the complexities of the modern supply chain landscape. GenAI’s ability to unlock hidden insights, optimize processes with laser focus, and foster proactive decision-making presents a compelling opportunity for businesses to achieve unprecedented efficiency, resilience, and sustainability levels.
However, realizing the full potential of GenAI hinges on a foundation of data-driven culture, well-governed high-quality data, and a commitment to responsible AI practices.