Keeping the Science in Data Science
By Tessa Jones, VP of Data Science Research & Development, Calligo
Under the umbrella of data science, machine learning (ML), artificial intelligence (AI), and statistical modeling are becoming ever more necessary to stay competitive. However, developing solutions that effectively integrate with the business and provide measurable value is no easy task. Many companies try and fail. Most data science focused tools in the market attempt to automate the development of models, removing the need for a data scientist at all. This leaves many companies with a difficult decision; take a risk with a product in the market and hope that it can produce something valuable or hire an expensive army of data scientists to build everything from scratch. Optimizing data science solutions is not achieved by removing data scientists from the process, but it can be optimized by focusing their time on specific elements of the data science process and leaning on automated technology to do the rest.
The evolution and advancement of technology is the hallmark of life in the twenty-first century. The applications we use on our computers, tablets, and phones fundamentally change the way we as humans engage with information, each other, and the broader world. With every new addition to the technological resources we enjoy, it’s easy to feel like we yield to artificial intelligence as its capabilities and influence grow. While there are robust conversations about both the benefits and drawbacks of technological progress, we see massive benefits come from new technology as we collect, manage, and make sense of data. In this data-rich landscape, it’s easy to be in awe of the computational power wielded by machines, but it is equally important to honor human intelligence and how it uniquely contributes to addressing problems. It behooves us first to understand the scientific process as it applies to solving business problems, then determine what components of that process should confidently be automated and which should stay rooted in human application. The scope of that inquiry is beyond this article, but we can pursue a similar investigation by looking at what outcomes we care about when embarking on a data science initiative.
Let’s consider, for a moment, what outcomes we care about. Generally, they can be broken down into the following graphic, which also demonstrates how the human to machine scale tips for each of the key outcomes. This framework highlights when, how, and to what extent machines can best be leveraged.
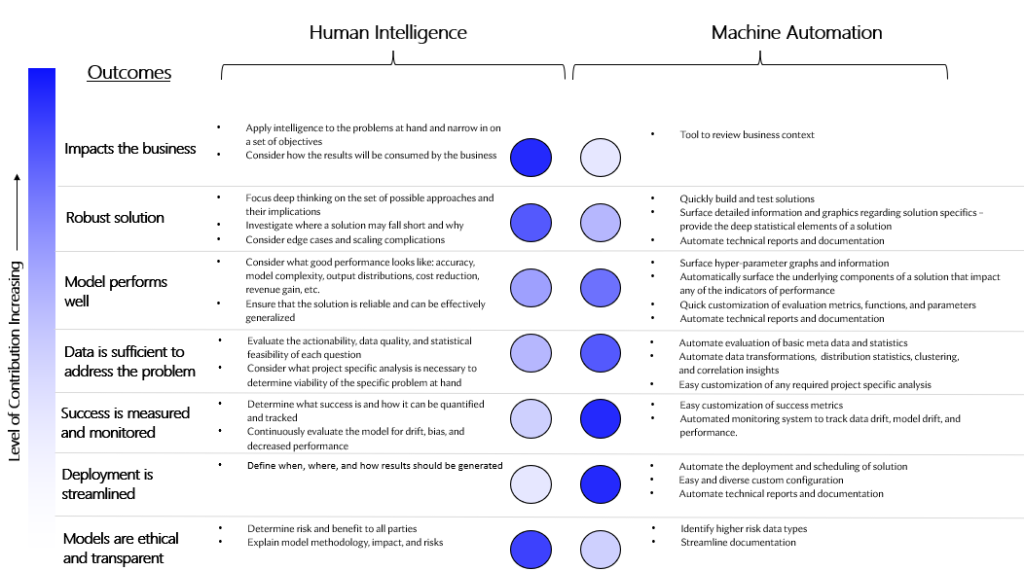
When evaluating this set of outcomes and the human/machine components, it is clear that while technology expedites and simplifies the execution of developing models, there is something special about the human mind and its ability to understand complexities that are difficult to quantify with machines alone. Perhaps the best example of this is technology’s inability to automate business acumen and discernment around business decisions: both of which are vital to successfully building and implementing a data science strategy. Within the foreseeable future, humans must incorporate their understanding of business processes and data to make decisions regarding what problems to solve, how to integrate solutions into the business process, and what data is necessary to develop solutions. Assessing the statistical application and nuance of a solution to optimize its power is also an inherently human contribution to data science development. In short, technology is only as powerful as those that leverage it. Understanding the delicate balance between the ingenuity of humans versus the computational abilities of machines within the development process allows us to produce high-value insights faster. With this approach, organizations can better determine where in the process their people should focus, and by contrast, where machines can contribute at a relatively low cost. Moreover, organizations can focus more on strategy and less on technical work, leading to more insightful, higher-impact business questions.
Technologies are not all created equally, and selecting the right technology requires a data scientist to consider the problem set, available data, and the computing power necessary to execute the job. While these aspects of a data science project are highly variable from one organization to the next, what remains constant is which components of the outcomes are impacted by leveraging machines and should rely on human input. No solution will balance the human input and machine automation perfectly, but a deeper understanding of how and why each is important could greatly improve the chances of success for any data science project.
Calligo unlocks the power of your data
We combine great minds in data science, privacy, security and engineering with leading machine learning, data analytics and cloud platforms to support the operational, customer-centric and revenue-generation aspirations of some of the world’s most ambitious and progressive organizations.
https://www.calligo.io